No one has missed that life is changing ever more rapidly!
As researchers, to unleash insights in this fast-changing world we need to be adaptative, but this doesn’t mean cutting corners!
The starting point of this article is our observation that, to guide new products design, some researchers are looking to carry on, or coming back to, using the ‘popular’ Monadic Quantitative test as the sole approach.
This test has been immensely popular for decades. However, for designing new products or reformulating products, it’s an incomplete approach that’s proven to be weak and, in many cases, even misleading.
Although most Consumer Insight Professionals in R&D have been successful in conveying this message to their Marketing and R&D partners, we still see Project Managers, Consumer Insight professionals, and sometimes also General Managers, suggesting the implementation of a monadic quantitative test as THE test for product testing and guiding (re)formulation.
So how does this “one size fits all” works?
The Monadic Quantitative test would include – on top of acceptability/liking questions – a list of “sensorial” questions to guide the product (re)formulation.
Whilst asking for preference or acceptability might be valuable, trying to find out why by asking direct questions doesn’t work…
This is because, when human beings say they like something, as soon as one asks why, the answer is rarely mirroring the real reasons for their liking! This doesn’t mean that they’re lying, but simply that they don’t know the real underlying reasons of the why they like something. Human beings don’t make their decisions rationally, because they are influenced by underlying cognitive bias and subconscious behaviours.
Product formulation/re-formulation, requires the ability to DECODE what consumers say they like into product features that REALLY drive product liking.
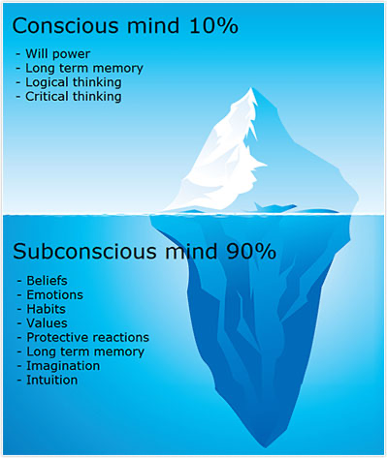
And this is the science of DECODING. What our industry calls “claimed drivers”.
For example: ‘’I like this Fabric conditioner because it brings me more softness” is a CLAIMED driver. It makes sense as consumers buy Fabric conditioner to soften their clothes.
The Monadic Consumer Quantitative tests are trying to understand the ‘‘why’’ by asking consumers additional questions on various attributes such as: how much they like the softness of the product? How soft are the clothes?… At this point, consumers give a subjective personal judgement with scores going often from “I like a lot, to I dislike a lot” or “too much to not enough”. But all those attributes are still “claimed” as they are statements made with their Conscious Mind. They are post-rationalising when answering the questionnaire, sometimes several days after having used the product.
This approach can help product developers when a product is far too sweet or salty or thick. However, it cannot go beyond the obvious, even when using the Penalty Analysis.
And that’s where the ‘One Size Fits All’ test reaches its limit…
Following the recording a Claimed Product Preference from Users/Consumers, how do we DECODE to the real WHY and guide product developers?
The name of the game is called “correlation”! And yes, it does require some statistical tools.
To DECODE what motivates those scores, a set of objective measurements is also required. Those data are often coming from sensory evaluation (but can also be clinical or instrumental). They’re reproducible,repeatable and describe the product features objectively (versus the subjective measurement from the consumer).
Statisticians then use correlation techniques to explain the subjective data (Consumer Quantitative Test – preferences/liking) with the objective data (Sensory/Instrumental Evaluation – product features intensities) and provide visibility on which are the product features that have an impact on the user/consumer preferences. So, nothing magical, but an analysis based on facts and data rather than opinions and gut feel.
In our Fabric Conditioner example (see below diagram), consumers discriminated between the different products by the level of Softness Perceived (top driver, on the left) and the temptation was to ask the product developers to increase the softness of our product to increase its Liking.
However, the Objective measurements (on the right) couldn’t perceive a softness difference between the products tested. But it perceived a difference in Thickness of the liquid (top driver, on the right) and has been able to correlate it with the Liking performance of the products.
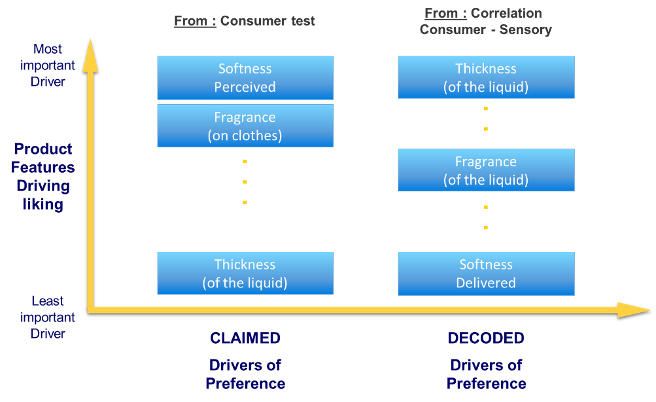
Diagram representing the relative importance of each driver on overall liking, for both claimed and decoded drivers.
This means that in a context where most brands propose a similar level of REAL softness (Softness Delivered) and where more active doesn’t provide more softness (plateau effect + increase cost) product cues (Thickness in this case) are key to the product developer to support perceived product performance (Softness Perceived) by the user. This also means (in this case) that the moment of truth is when the product user uses the product rather than the result on dry clothes.
Of course, no consumer will give you this explanation in a quantitative test even with a strange question like “how important is the liquid thickness?”!
Once the Development team understands that the thickness of the liquid significantly cues the perceptions of softness, the next challenge is to understand how consumers check that cue for reassurance. In this instance, in some countries, it already starts in the supermarket where consumers shake the bottle and the sound of the liquid will influence their purchase behaviour. Why? Because unconsciously the lower the sound, the thicker the liquid. Of course, once again no consumer will give you this explanation in a quantitative test. Imagine the surrealist question like “rate the sound when shaking the bottle”? That insight can only be unleashed thanks to qualitative research.
Getting to that connection: Softness – Thickness – Sound is the result of several pieces of research, not just One Size Fits All test.
As mentioned at the start of this article, the world is changing, and we need to adapt.
Adapt does not mean take the same test but do it digitally!
Adapt means: embrace diversity of tools, bring product developers and marketers closer to the consumers, assess prototypes earlier and trust qualitative approach.
The challenge for researchers is to adapt and bring insights that lead to business decisions and product developments – this is the whole approach behind the growing Agile methods. Easier said than done of course!
Share your experience – either you still get product insights from Monadic Quantitative tests or you have moved away or even found a mix… let’s learn from each other and carry on the dialogue.
We would like to thank Kartin Costa (Consumer Insights Director, Reckitt Benckiser), Nieke Gerritsen (Global Director Consumer Technical Insights, Unilever), Cecile Lorenzo (R&I Director, Danone UK, BE, Nl) and Juliana Oliveira (Head of Product & Consumer Insights, Danone Latam) for their invaluable contributions to this article.